Engineering-genes Based Modelling of Biochemical Reaction Networks
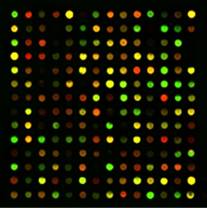
Researchers: Mr Padhraig Gormley and Dr Kang Li
Introduction
Only in recent years, systems biology or molecular systems biology have emerged as new promising approaches in biology to build better predictive models of diagnosis, prognosis, and therapy, and to unravel the basic dynamic processes, feedback control loops, and signalling network and regulation mechanisms at the cellular level of the living creatures. In particular, high-throughput genomic microarray and proteomic technology allow simultaneously monitoring of the activity and temporal relations of numerous genes and the concentrations of proteins and metabolites (see Figure 1), thus allows the study to infer network connections between particular genes that interact with each other and conversely, genes that are entirely unrelated. By inferring these gene regulatory networks we can gain insight into the function of the underlying biological processes and uncover previously unknown relationships between molecular species within the cell. It can be forecasted that accurate modelling of these molecular processes can provide an inexpensive method for testing molecular targets and hypotheses relating to many types of genetic diseases such as cancer.
However, in system biology, investigators are confronted with the high dimensionality problem, i.e. each data sample could be defined by hundreds or thousands of measurements that might be concurrently obtained. For example, it is typically estimated that the human genome consists of up to 25,000 genes. Attempting to extract meaningful expression patterns, or to infer related genes and pathways, from small sets of (time-series) data in such high dimensional space is incredibly difficult.
The aim of this project is to use engineering-genes based method to reverse engineer the network structure and parameters of the hidden system from the gene expression data.
Methods and Results
In systems biology, molecular interactions are typically modelled using white-box methods, usually based on mass action kinetics. Unfortunately, problems with dimensionality can arise when the number of molecular species in the system is very large, which make the system modelling and behaviour simulation extremely difficult or computationally too expensive. As an alternative, the work carried out first is to apply a novel two stage method to identify a black-box model of the MAPK signal transduction pathway and the Brusselator using noisy data of different size. This type of method creates a simple linear-in-the-parameters model using regression of data, where the output of the model at any time is a function of previous system states of interest. One of the main objectives in building black-box models is to produce an optimal sparse nonlinear one to effectively represent the system behaviour. Simulation results confirm the efficacy of the black-box modelling method which offers an alternative to the computationally expensive conventional approach (see Figures 2 and 3).
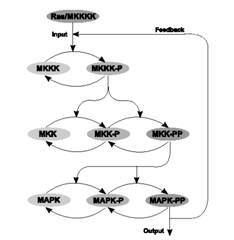
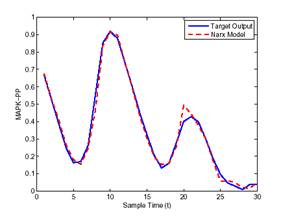
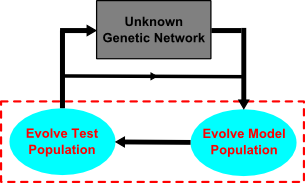
Next, the research is focused on modelling of signalling pathway using eng-genes based reverse engineering approach, where the structure and parameters of nonlinear models are reverse engineered. Firstly, the model structure is selected from a pool of engineering genes – fundamental nonlinear functions relating to the system behaviour extracted ‘a priori’ bio-chemical knowledge. The final structure and model parameters are then searched using a coevolutionary approach, which consists of two separate populations of candidate models and intelligent tests to perturb the models (see Figure 4). These two populations are evolved in tandem to improve the modelling accuracy and speed of convergence.
Acknowledgements
This work has been partially supported by the Engineering and Physical Sciences Research Council (EPSRC) for funding this project (GR/S85191/01), and Department for Employment and Learning (Northern Ireland).
Publications
- P. Gormley, K Li, G. W. Irwin, “Modelling molecular interaction pathway using a two-stage Identification Algorithm”, Systems and Synthetic Biology (accepted), 2008.
- K. Li, X. Li. G. Irwin, G. He (editors), Life system modelling and simulation. Lecture Notes in Bioinformatics, Springer-Verlag GmbH. LNBI 4689, 2007.
- P. Gormley, K. Li, G. W. Irwin, “Modelling the MAPK Signalling Pathway using a 2-Stage Identification Algorithm”, Life System Modeling and Simulation, Lecture Notes in Bioinformatics, Springer-Verlag GmbH, Volume 4689, 2007. 480-491.
Keynote speech
- G. W. Irwin, K. Li, “Computational Intelligence for Data Modelling with Life Science Applications”, International Conference on Life System Modelling and Simulation, Shanghai, September 14-17, 2007.